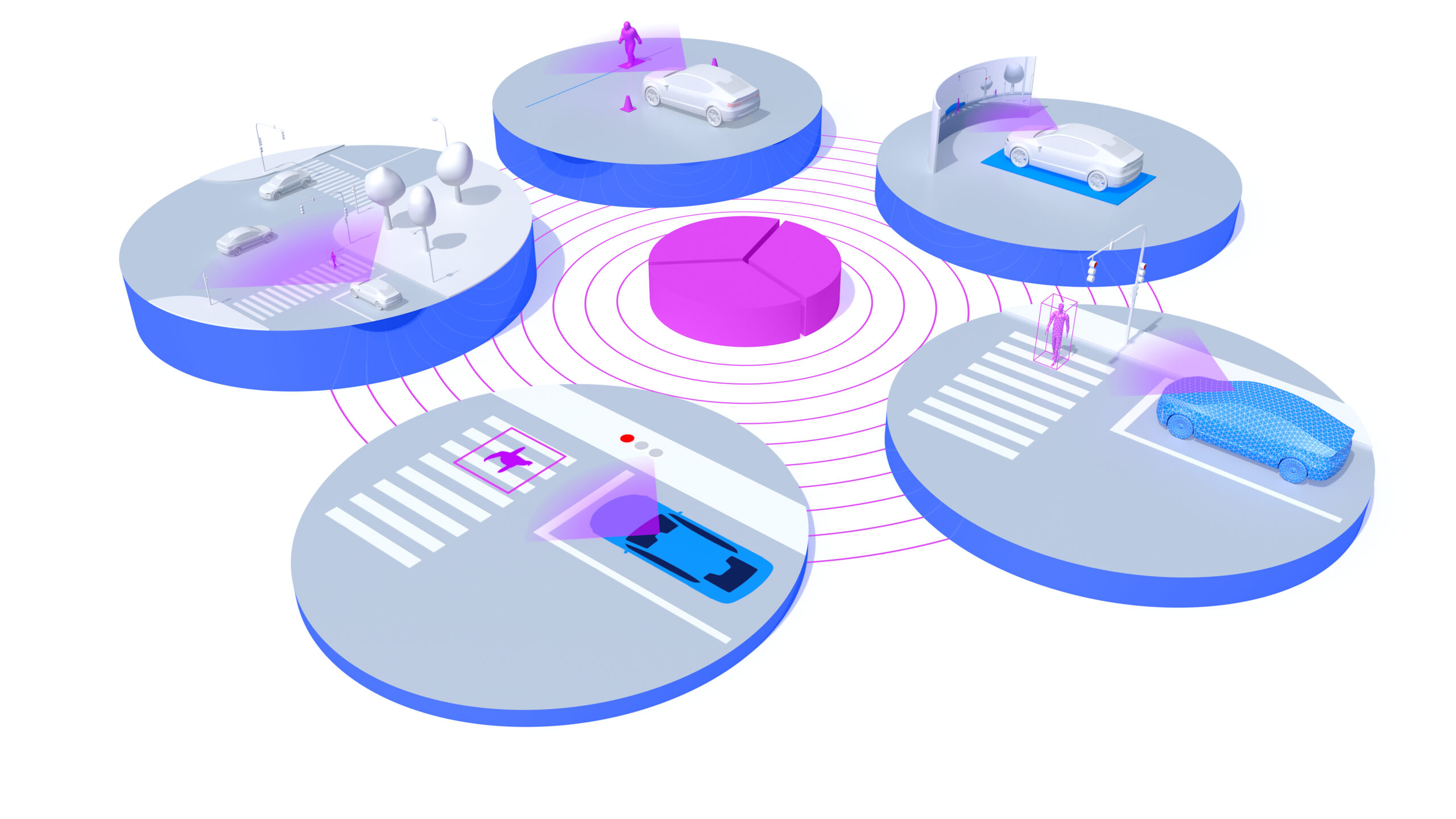
How can an automated vehicle get into a critical situation, and how can this be prevented? Under what setting does a wind turbine produce high amounts of energy with low wear at the same time? Such and similar questions can be answered by data-driven modeling.
Basically, experiments are parameterized for this purpose, performed during the development process on digital prototypes or even on the physical system. The results of the measurements can be linked to the input data. In this way, development teams can investigate how great the influence of the different input parameters is.
In addition, modelling allows further critical points to be predicted. With such predictions, the questions posed at the beginning can be answered. But this can tie up a lot of resources, even if the tests are performed virtually but manually.
AI as a key to success
A software approach developed by IAV combines algorithms and AI to generate targeted test scenarios and check whether a specific event or system state occurs. This process runs iteratively and adapts autonomously to the previously unknown system and its characteristics.
In this way, the parameter space to be considered can be narrowed down, which results from the value ranges of all system parameters to be considered. The software also indicates the probability with which an event can occur, thus additionally creating a measurable basis for the release decision of safety-critical systems.
By using artificial intelligence, the methodology goes beyond the approaches of classical optimization and design of experiments. Characteristic of this method is the use of probabilistic metamodels and iterative, adaptive design of experiments (Adaptive Importance Sampling).
Application example: Automated driving
In automated driving, a vehicle maneuvers autonomously through a city center. In doing so, it is supposed to avoid a collision with pedestrians or other road users in a wide variety of situations. For such a scenario, 20 or more parameters can easily be defined here. These can be, for example, scenario parameters or function parameters. Scenario parameters can be different speeds or distances to individual objects. Different sensor ranges or reaction times of the
of the automated vehicle are examples of function parameters.
In a first step, a test plan is created that distributes concrete measurement points throughout the test space. The first batch of experiments is performed on the system under investigation. For the given example, a parameterization of the simulation is performed: vehicles drive virtually through the created scenario. Finally, the output of each concrete scenario is stored. This data can be used further on to train probabilistic metamodels and predict unknown scenario outputs.
Based on these metamodels, the uncertainty of the generated model and the relevance criterion, the software places new points in the parameter space at relevant
positions. Thus, with each iteration, the model quality, i.e. the accuracy of the predicted results, is improved in a targeted manner. For points with similar measurement results and no further gain in knowledge, the AI also does not perform any further tests. However, if the system ratio changes quickly or in critical directions, this is an indication for the algorithm of a potentially important situation - the software then continues to search specifically in this range of values, thereby increasing the understanding of the overall system with each iteration.
Transfer to other systems
The method was originally developed for automated driving, since until now there have been no methods to limit the parameter space to be considered for validation and at the same time to provide information about the residual uncertainties required for approvals.
For validation and, at the same time, to provide a statement about the residual uncertainties required for approvals. However, the approach can also be used wherever efficient methods for modeling are required for the development or release of complex systems - for example in the energy sector, robotics or even in aviation.
The software thus has the potential to make development processes much more efficient and to reduce large-scale testing. For example, if developers wanted to test 20 parameters with three values each - the highest, the middle and the lowest - taking into account all conceivable possible combinations, they would need several billion tests. Also, trying to manually select meaningful test cases is hardly feasible in a reasonable time frame given the large number of influencing parameters.
With the help of the new methodology, results can be achieved much faster. For a complex example in a parameter space with 20 parameters, the IAV software was already able to exclude 98 percent of all possible tests after 5,000 trials - this means that further investigation focuses on the remaining two percent of the parameter space.
Conclusion
The AI-based method shown offers new possibilities for designing technical systems and software and for testing their functional reliability. It thus also efficiently relieves the burden of releasing and validating a final system. For this purpose, only the parameter space to be tested, and the test target have to be defined.
The rest is done automatically and highly efficiently. In this respect, the method presented is an essential building block for developing and validating complex systems with little time and personnel effort in the future.
Contact:
Mike Hartrumpf
Product Owner in the team of Simulation & Modeling at IAV
mike.hartrumpf@iav.de
linkedin.com/in/mike-hartrumpf