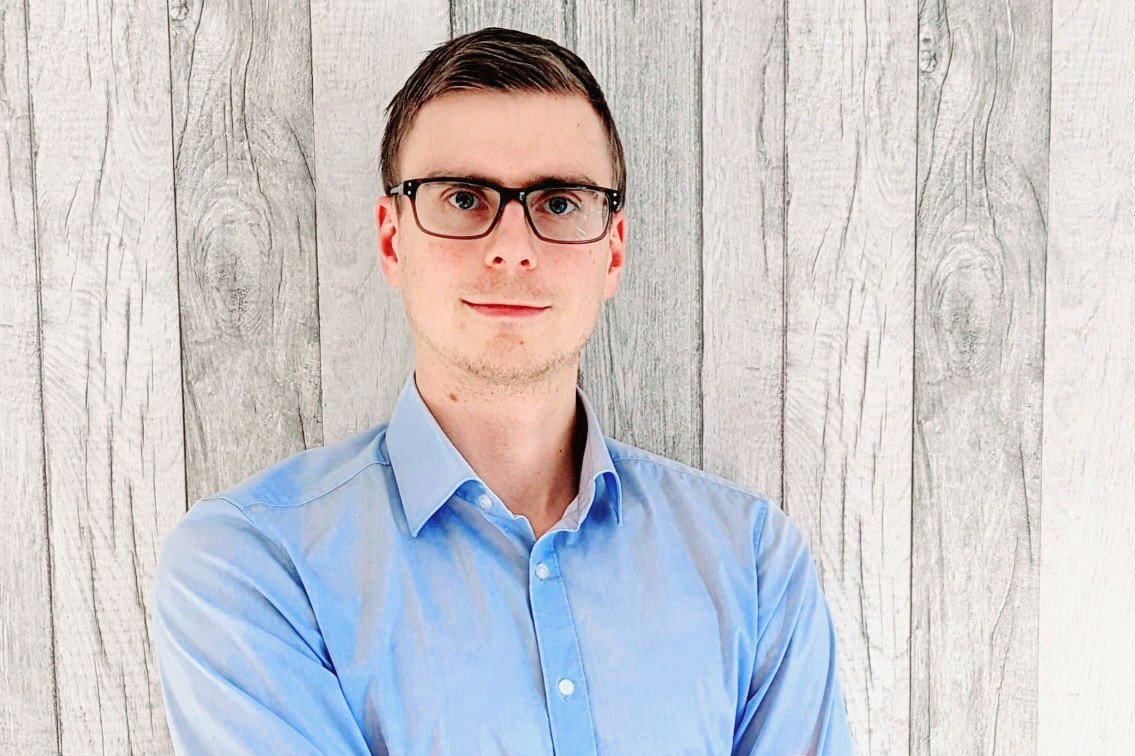
AI has long since found its way into engineering. Why are large language models like the technology behind ChatGPT particularly important for engineering?
Large language models are important because they can process natural language and generate new content. In engineering, a lot of work is done with language, be it requirements, software code or test cases. These AI models help to increase efficiency, shorten development cycles and improve quality.
How does artificial intelligence support developers in turning ideas from proof of concept into concrete added value for the economy and society?
AI developers face several hurdles. They need to develop a stable technological core that provides the desired accuracy for all applications. They should also consider cost optimisation, IT and data security, and regulatory requirements. Another issue is the user interface. While ChatGPT offers a user-friendly interface, this is often not enough in engineering. Developers need tools that are seamlessly integrated into their daily work processes.
How does working with artificial intelligence actually work?
Let's say I'm writing a book and want ChatGPT to write the next chapter based on my previous ideas. I won't adopt the result 1:1, but I will adapt it. If I want to change a sentence, it would be easier to do so directly in a text editor. The situation is similar in the automotive sector. Developers need a user interface that is adapted to their work processes and where they can interact with results as needed to work efficiently with AI tools. AI can be used to check project requirements against many formal criteria. If necessary, the AI can also make suitable suggestions for corrections, which can be accepted or revised.
In this context, IAV has coined the terms ‘AI-infused company’ and ‘AI-infused engineering’. What do they mean?
The term AI-infused company refers to the integration of artificial intelligence into all areas of the company. One example is the in-house chatbot, which is familiar with internal company information and works like ChatGPT. This Wisdom Bot can be used by all employees for everyday questions about responsibilities within the company or for IT problems and much more.
AI infused engineering refers to the use of AI in technically demanding issues, i.e. where our company's greatest added value lies. There are fewer out-of-the-box solutions here, which is why our company develops its own AI solutions to achieve added value in the market.
In this context, AI-infused generally means that every step of the development process and the entire business unit consider where AI can already make a meaningful contribution today. It's about using existing low-threshold AI tools like ChatGPT and others to increase efficiency and productivity or create your own solutions.
We used to often wonder whether a step could be automated. The hurdle was high because you had to write a script and needed someone who could do that. The threshold is now lower. AI infused means considering every step: Can I use AI sensibly here to achieve automation?
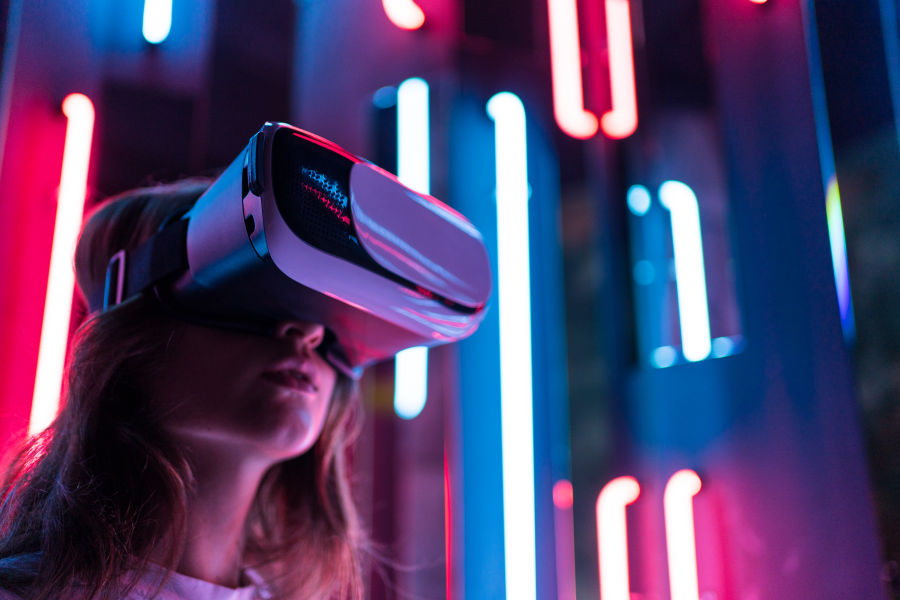
… and that's why IAV has developed an entire AI platform?
Exactly, IAV has developed an extensible platform that combines many useful AI tools to address various engineering challenges. This platform is designed so that more and more modules and functionalities can be added over time.
The platform currently covers topics such as requirements management, creating test cases, implementing software code, e.g. using Code Pilot, and handling the complexity of project data. For example, complex dependencies between functions can be displayed and made interrogable by a chatbot to extract information and examine dependencies.
Hundreds of thousands of requirements are written for the development of vehicles. With the Requirements Quality Checker, we have developed an AI service to accelerate the recording of requirements and to increase the quality of the data. This service checks project requirements for numerous formal criteria and can also correct the requirements accordingly if necessary. Within a month, around 35,000 requirements from over 250 users are processed at IAV.
The platform provides a unified basis and a unified backend, which reduces many of the challenges mentioned earlier. The idea behind this is to create a flexible and expandable solution that covers various use cases in engineering and can be continuously developed.
Where do we start in the tool chain?
Typically, we start with the requirements, which we create first. Such requirements must meet high quality standards, as errors at the beginning of the chain can have many effects. This is where AI supports us. For example, we currently check the consistency of the requirements, whether there are contradictory requirements or whether they are formally written down properly. Later in the chain, when requirements are changed, we can use the platform to help analyse the effects of these changes and to recreate the test cases accordingly.
“The biggest challenge, however, is to enable users to use AI sensibly and to be aware of its potential and limitations. It is important that technology does not prevent us from using our heads and always asking critical questions.“
Christian Nabert – AI expert at IAV
Does this mean that if I use the AI solution for requirements management, I can derive test cases directly from it?
Yes, together with the architecture, the requirements form the starting point for test cases. Each requirement is checked by test cases. Test specifications can be derived with the help of AI. We have already realised time savings of over 10% with this AI-supported creation of test specifications, but the potential is far from exhausted. If we combine large language models, i.e. AI-based language models, with technologies such as knowledge graphs, we can reliably generate correct test cases.
Where are the limits of AI in engineering at the moment?
AI reaches its limits when it comes to the complete automation of complex, overarching activities. It cannot develop complete cars based on general requirements and has difficulties with overarching tasks that require different roles. In addition, safety-critical applications are challenging because they require a high degree of precision and reliability, for example in the recognition of traffic lights.
To be able to use AI comprehensively, we have to make all the necessary data available. In established structures, this often requires adjustments. It is also essential that the data is of sufficient quality and is not contradictory. The biggest challenge, however, is to enable users to use AI sensibly and to be aware of its potential and limitations. It is important that technology does not prevent us from using our heads and always asking critical questions.