Why PLP?
Car manufacturers want to assess the condition of their car fleets in the field in order to estimate the risk of failure of individual components. Precise knowledge of the ageing condition enables needs-based maintenance and thus reduces the number of failures.
Forecasting failure rates into the future has further advantages. For example, future warranty costs can be estimated and the stocking of spare parts can be better planned. The patented IAV method of probabilistic service life forecasting is aimed precisely at these issues.
The method enables the prediction of future failures by analysing vehicle-specific mileages and current failure statistics. The core of the method is the use of probabilistic models that combine the advantages of machine learning and statistics. This allows all uncertainties in the data to be taken into account and visualised for the analysis.
Complete automation eliminates the need for time-consuming data cleansing and model customisation steps, meaning that simple, scaled use is also possible for non-experts. The process has been implemented in Microsoft Azure as a machine learning pipeline and validated across many use cases. The implementation is currently running as a software-as-a-service application in AWS.
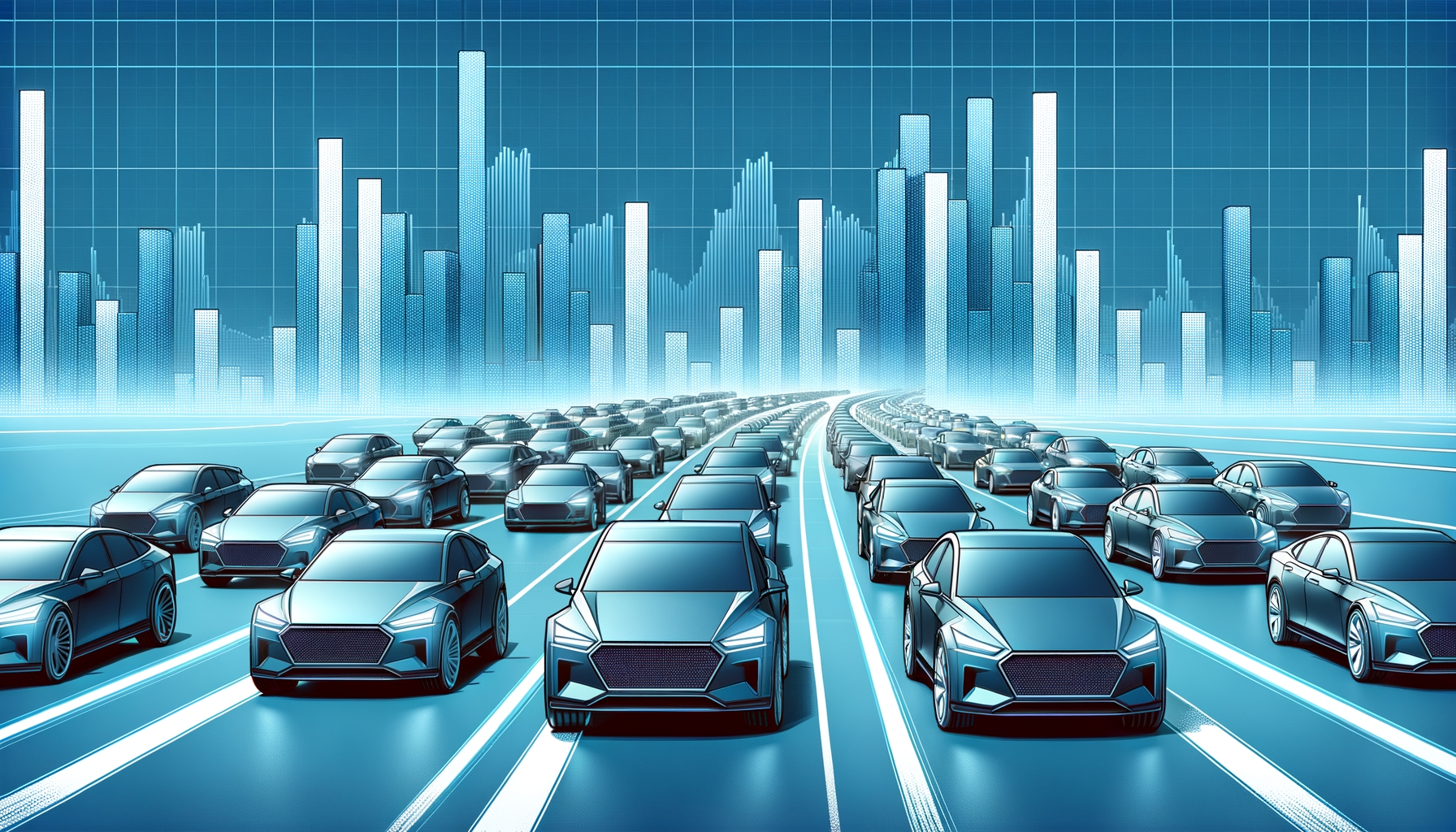